In the modern industrial landscape, efficiency and sustainability are paramount. One area where these goals converge dramatically is in the optimization of piping systems. Piping system optimization is crucial for industries ranging from oil and gas to water treatment facilities. The application of Machine Learning (ML) in piping system optimization presents a groundbreaking opportunity to enhance system efficiency, reduce costs, and improve environmental sustainability.
Why Optimize Piping Systems with Machine Learning?
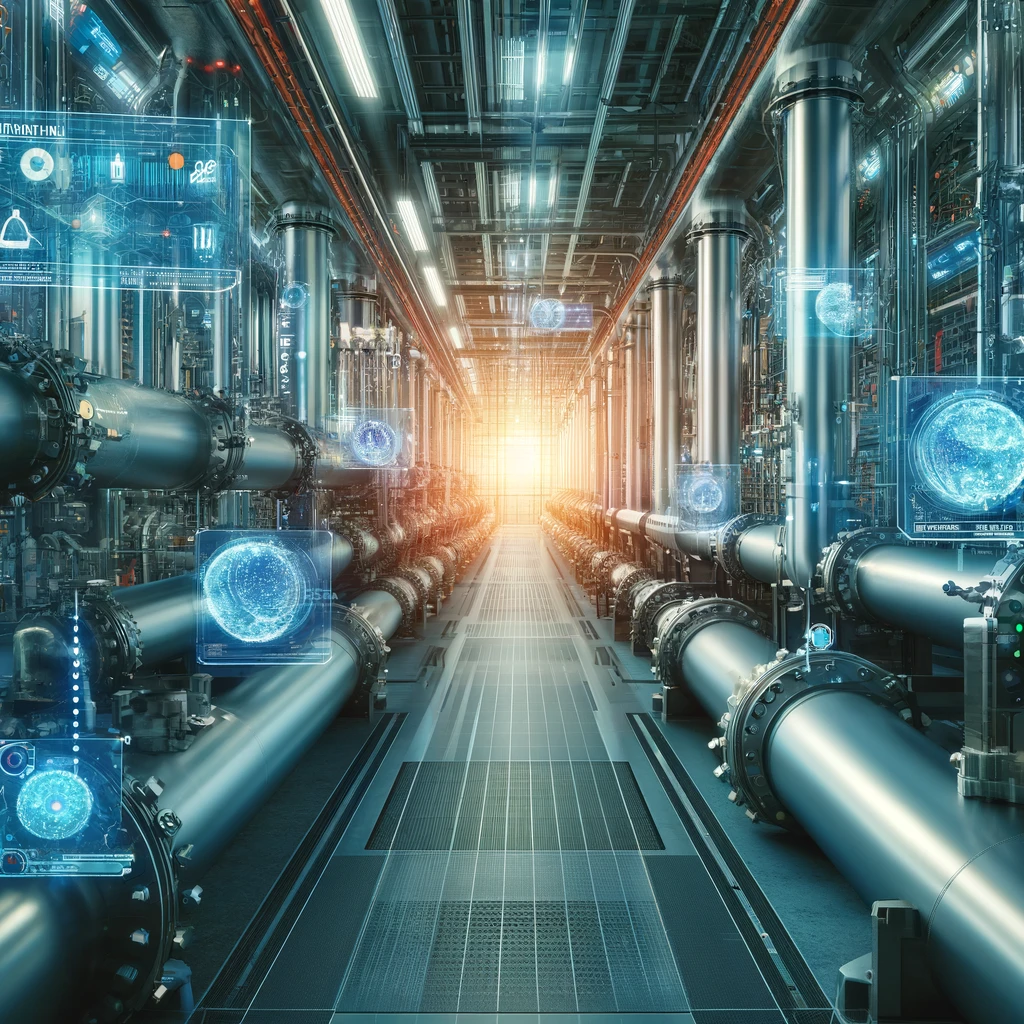
Piping systems are the arteries of many industrial sectors, carrying fluids necessary for various processes. Optimizing these systems helps in reducing energy consumption, minimizing operational costs, and extending the infrastructure’s lifespan. Machine Learning leverages data-driven insights to predict system behaviors, enhance decision-making, and identify optimization opportunities in real-time. Implementing ML in piping system optimization can lead to significant improvements in performance and cost-efficiency.
The Role of Machine Learning in Piping System Optimization
Machine Learning can transform piping system optimization through several key applications:
Predictive Maintenance
ML algorithms analyze historical and real-time data to predict potential failures before they occur. This predictive capability ensures that maintenance can be scheduled at the optimal time to prevent downtime and expensive repairs.
Fluid Dynamics Simulation
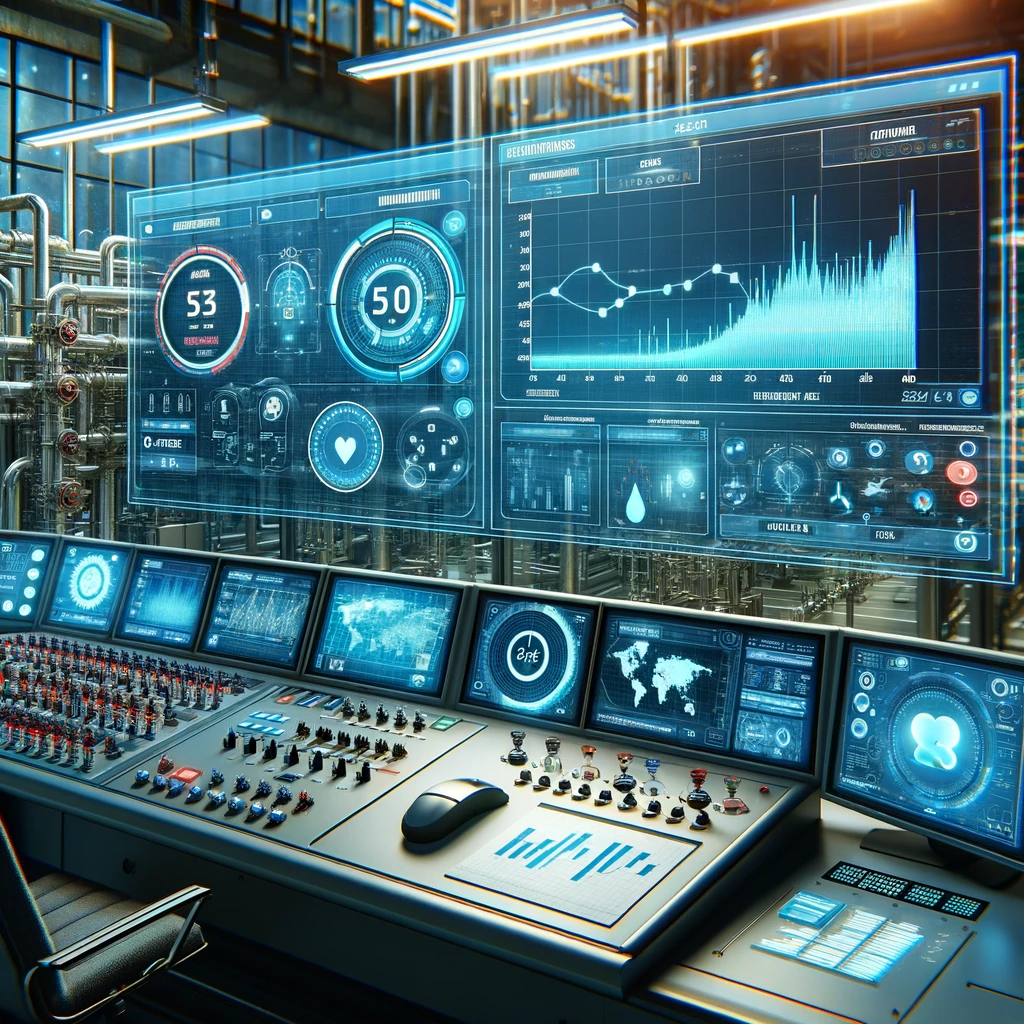
ML models can simulate fluid dynamics within the pipes, predicting how different variables affect flow rates, pressure drops, and energy consumption. This simulation helps in designing more efficient piping systems and optimizing existing setups.
Leakage Detection
Machine Learning can enhance the sensitivity of leakage detection systems in pipelines. By analyzing pressure and flow data, ML models can identify even minor leaks, which might go unnoticed by conventional monitoring systems.
System Configuration
ML algorithms can recommend changes to the configuration of a piping system, such as adjusting pump speeds or valve positions to optimize flow and minimize energy use.
Case Studies
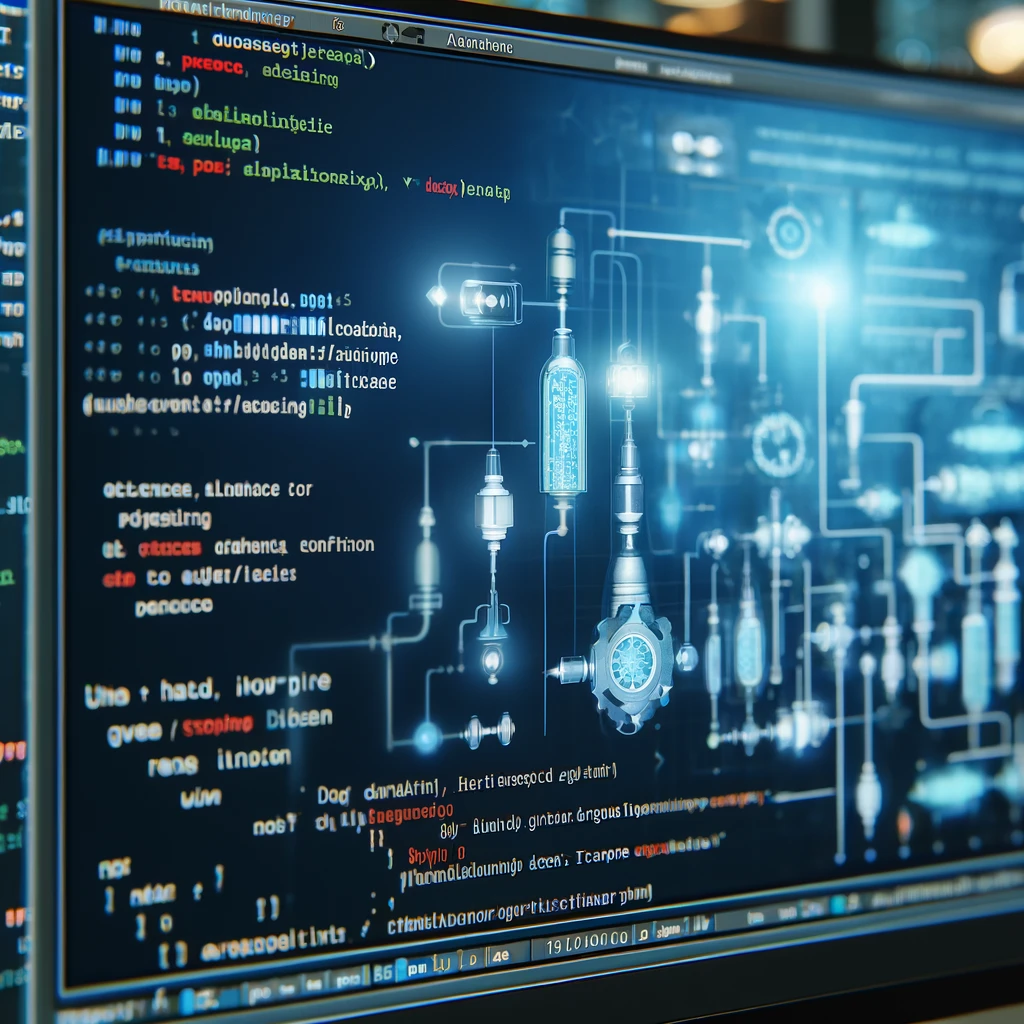
Several industries have successfully implemented piping system optimization using Machine Learning:
- Oil and Gas: Major oil companies have used ML to optimize the flow of oil through vast pipeline networks, significantly reducing pump energy usage.
- Water Utilities: Water treatment plants have implemented ML to detect leaks and optimize the treatment process, ensuring efficient water distribution and conservation.
Future Trends
As Machine Learning (ML) technology advances, the potential for its application in piping system optimization is poised for exponential growth and sophistication. Here are several key future trends that may shape this field:
- Autonomous Piping Systems: Future developments are likely to introduce fully autonomous piping systems capable of self-optimizing in real-time. These systems would use ML to continuously analyze performance data and automatically adjust parameters without human intervention, thus enhancing efficiency and reducing operational costs.
- Advanced Predictive Analytics: Enhanced predictive analytics will allow for more precise forecasting of system failures, maintenance needs, and operational inefficiencies. These analytics will utilize deeper and more complex neural networks to process vast datasets, leading to significantly improved accuracy in predictions and decision-making processes.
- Integration with IoT Devices: The integration of the Internet of Things (IoT) with ML will enable more comprehensive monitoring and control of piping systems. Sensors will collect data on various aspects of the system’s operation, which ML algorithms can use to optimize performance, predict equipment failures, and schedule maintenance automatically.
- Energy Optimization: As energy efficiency becomes increasingly crucial, ML will play a pivotal role in minimizing energy consumption in piping systems. Algorithms will optimize the flow rates and pressures across the network to reduce pump usage and energy costs, contributing to more sustainable practices.
- Customization and Scalability: ML algorithms will evolve to be more customizable and scalable, catering to the specific needs of different industries and the sizes of their piping systems. This adaptability will allow organizations to implement solutions that are precisely tailored to their operational requirements and constraints.
- Enhanced Safety Protocols: ML will also enhance safety measures within piping systems, particularly in industries handling hazardous materials. By better predicting and preventing leaks and failures, ML can significantly mitigate risks associated with spills and explosions.
Conclusion
The integration of Machine Learning into piping system optimization is a transformative shift that continues to reshape various industries. This is not merely a trend but a fundamental evolution in how piping systems are managed and optimized. As companies increasingly prioritize sustainability and cost-effectiveness, the role of ML in enhancing the efficiency, safety, and reliability of these systems will undoubtedly grow. This shift promises smarter, more efficient operations with reduced environmental impact, heralding a new era of industrial operation where technology and operational needs converge seamlessly.